Overview
Open Source, Distributed Machine Learning for Everyone
H2O is a fully open source, distributed in-memory machine learning platform with linear scalability. H2O supports the most widely used statistical & machine learning algorithms including gradient boosted machines, generalized linear models, deep learning and more. H2O also has an industry leading AutoML functionality that automatically runs through all the algorithms and their hyperparameters to produce a leaderboard of the best models. The H2O platform is used by over 18,000 organizations globally and is extremely popular in both the R & Python communities.
Key Features of H2O
- Leading Algorithms
- Access from R, Python, Flow and more…
- AutoML
- Distributed, In-Memory Processing
- Simple Deployment
Algorithms developed from the ground up for distributed computing and for both supervised and unsupervised approaches including Random Forest, GLM, GBM, XGBoost, GLRM, Word2Vec and many more.
Use the programing language you already know like R, Python and others to build models in H2O, or use H2O Flow, a graphical notebook based interactive user interface that does not require any coding.
H2O’s AutoML can be used for automating the machine learning workflow, which includes automatic training and tuning of many models within a user-specified time limit. Stacked Ensembles will be automatically trained on collections of individual models to produce highly predictive ensemble models which, in most cases, will be the top performing models in the AutoML Leaderboard.
Learn MoreIn-memory processing with fast serialization between nodes and clusters to support massive datasets. Distributed processing on big data delivers speeds up to 100x faster with fine-grain parallelism, enabling optimal efficiency without introducing degradation in computational accuracy.
Easy to deploy POJOs and MOJOs to deploy models for fast and accurate scoring in any environment, including very large models.
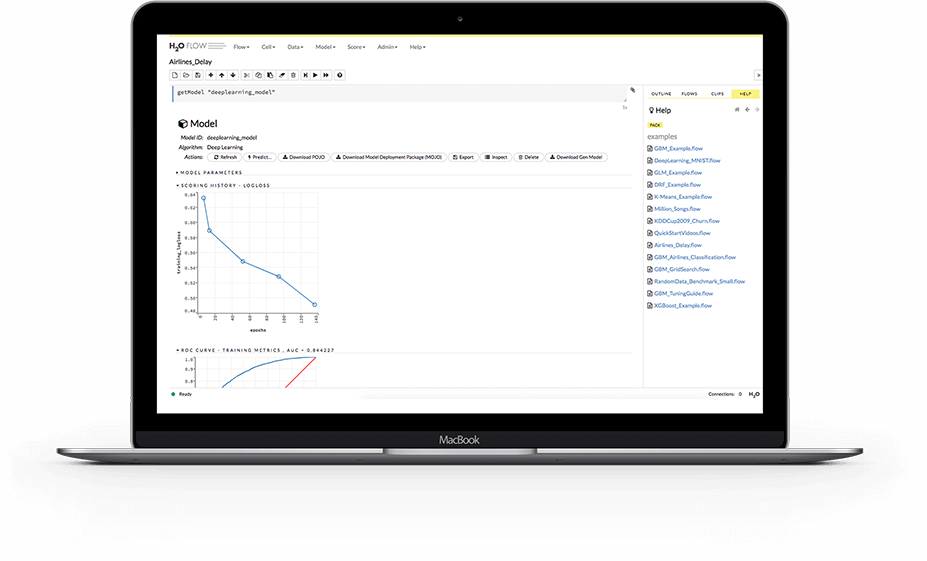
How it Works
Enterprise Data
H2O works on existing big data infrastructure, on bare metal or on top of existing Hadoop, Spark or Kubernetes clusters. It can ingest data directly from HDFS, Spark, S3, Azure Data Lake or any other data source into it’s in-memory distributed key-value store.
Distributed, In-Memory Machine Learning
H2O takes advantage of the computing power of distributed systems and in-memory computing to accelerate machine learning using it’s industry parallelized algorithms which take advantage of fine grained in-memory mapreduce..
Seamless Deployment
Quickly and easily deploy models into production with Java (POJO) and binary formats (MOJO). In addition, H2O models can be productionized in a host of different ways as listed here.
Featured Use Cases
Related Case Studies
Adam Sullivan
Director, Change Healthcare
"H2O has been the driver for building models at scale. We are talking about billions of claims. You can't do this with standard off the shelf open source techniques. "
Watch the VideoIntroduction to Machine Learning with H2O Tutorial
In this tutorial for the H2O platform, you will learn how to use H2O's GLM Random Forest, GBM Models, and grid search to tune hyperparameters for a classification problem.
We will be using a subset of the Freddie Mac Single-Family dataset to try to predict whether or not a mortgage loan will be delinquent using H2O's GLM, Random Forest, and GBM models. We will go over how to use these models for classification problems, and we will demonstrate how to use H2O's grid search to tune the hyper-parameters of each model.
Learn More
H2O Download Options
H2O works with R, Python, Scala on Hadoop/Yarn, Spark or your laptop.
H2O is licensed under the Apache License, Version 2.0